E-commerce Analytics for Data-Driven Decision Making: 7+ Key Steps
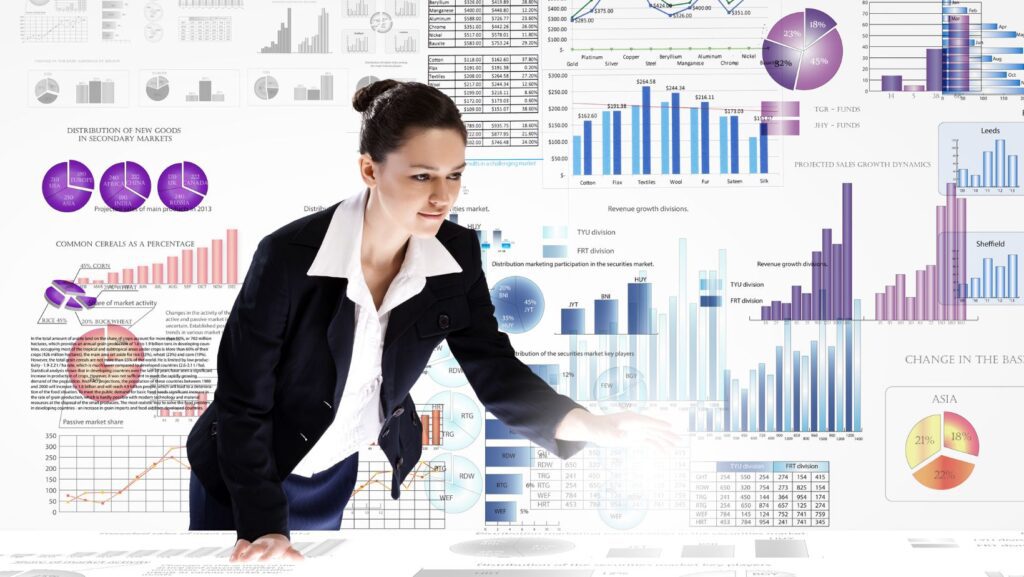
Businesses must harness the power of data and analytics to make wise decisions and keep a competitive edge in the quickly changing and fiercely competitive world of e-commerce.
The purpose of the article is to highlight the crucial part that data-driven decision-making plays in businesses’ success.
It will examine the numerous analytics programs and KPIs that are crucial for gauging and improving performance.
Understanding Key E-commerce Metrics
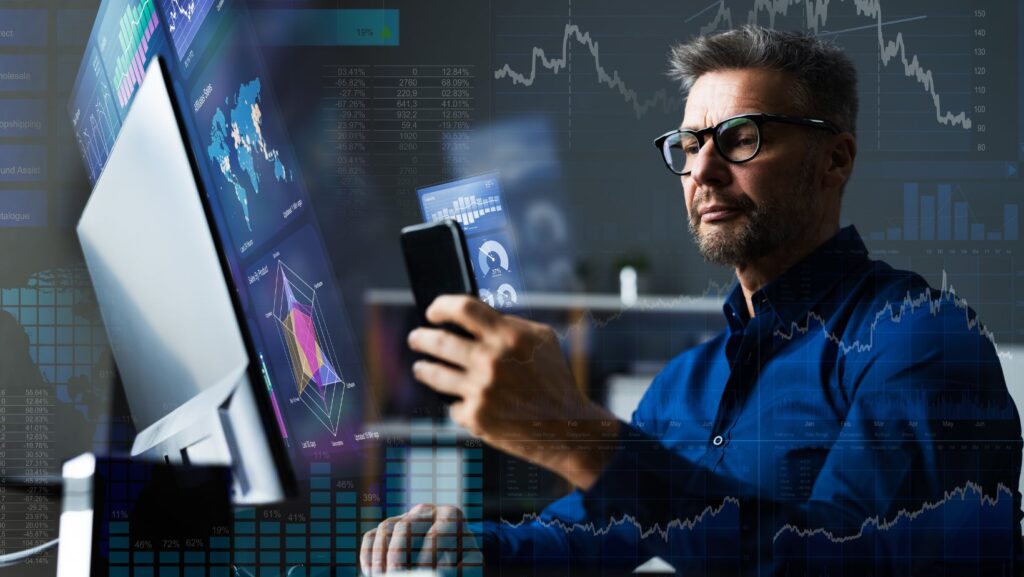
You must have a thorough awareness of the important metrics that function as success indicators in order to make wise decisions and maximize the performance of your e-commerce business.
Web traffic and user behavior, sales success, and customer involvement can be used as broad categories to group these indicators.
Each category offers insightful information on many facets of your company, enabling you to take data-driven decisions that promote development and expansion.
Metrics on web traffic and user behavior provide insight on how visitors use your website, exposing potential problems and openings for optimization.
These indicators enable you to evaluate the general success of your website in luring, holding, and retaining users, which has a direct bearing on your conversion rates and bottom line.
Sales performance measurements give you insights into revenue creation, profitability, and overall success by focusing on the financial components of your e-commerce business.
Recognize trends, identify problem areas, and seize development opportunities because of these analytics.
Customer engagement metrics show you how successfully you’re relating to your clients and creating lasting relationships.
These indicators are crucial for tracking consumer satisfaction, loyalty, and advocacy because they all affect how successfully and sustainably your e-commerce business operates.
Web Traffic and User Behavior
Bounce rate: This statistic shows the proportion of visitors who abandon your website after just seeing one page. A high bounce rate may indicate problems with the layout, usability, or content of your website, which can turn off potential clients.
Conversion rate: The proportion of users who carry out a desired activity, like making a purchase, is measured by this metric. You may improve sales without spending more on marketing by optimizing your conversion rate.
Average session duration: This metric shows how long users spend on your site, providing insights into their engagement levels. Longer sessions suggest that users find the content valuable and are more likely to make a purchase.
Sales Performance
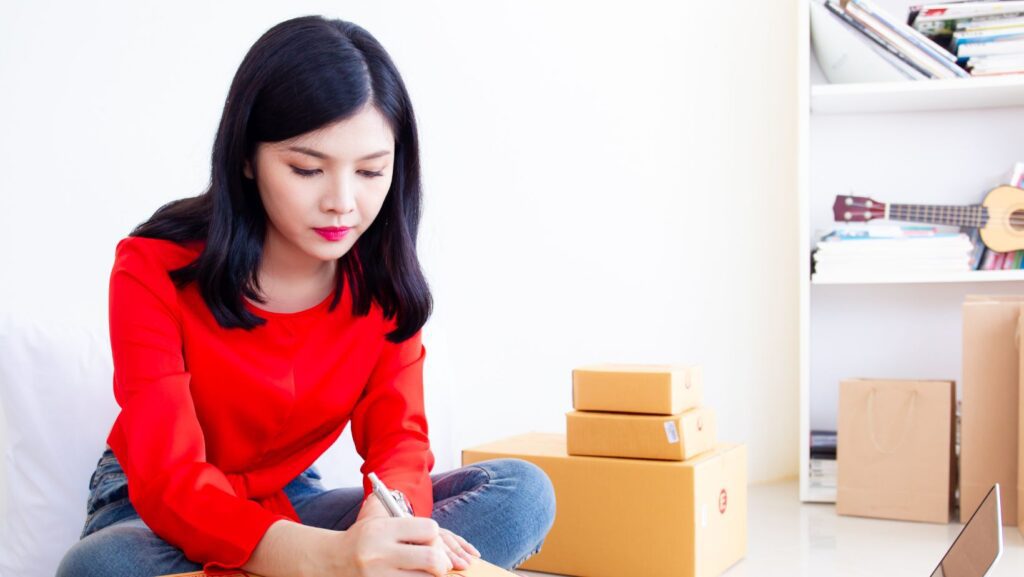
The overall revenue your e-commerce store generates is a critical statistic for assessing the performance of your company as a whole. You can spot possible problems and areas for growth by keeping an eye on revenue trends.
The average amount spent on each transaction is measured by average order value, or AOV. Strategies like upselling, cross-selling, and supplying bundled products will boost AOV and as a result profitability.
The gap between revenue and cost of goods sold is known as gross margin. You may better understand the profitability of specific items and determine appropriate pricing by keeping an eye on gross margin.
- Revenue
- Average order value (AOV)
- Gross Margin
Customer Engagement
The percentage of customers that make multiple purchases is shown by the repeat customer rate.
Strong client loyalty is indicated by a high proportion of repeat business, which can result in a company’s long-term success.
Client lifetime value (CLV) is an estimation of the whole revenue a client will bring in during their association with your company.
You may maximize the return on your marketing investments by concentrating on growing CLV.
When customers are asked how likely they are to suggest an ecommerce site to others, the Net Promoter Score (NPS) is calculated.
Strong brand advocacy, which can spur organic development via word-of-mouth recommendations, is indicated by a high NPS.
Analytics Tools and Platforms
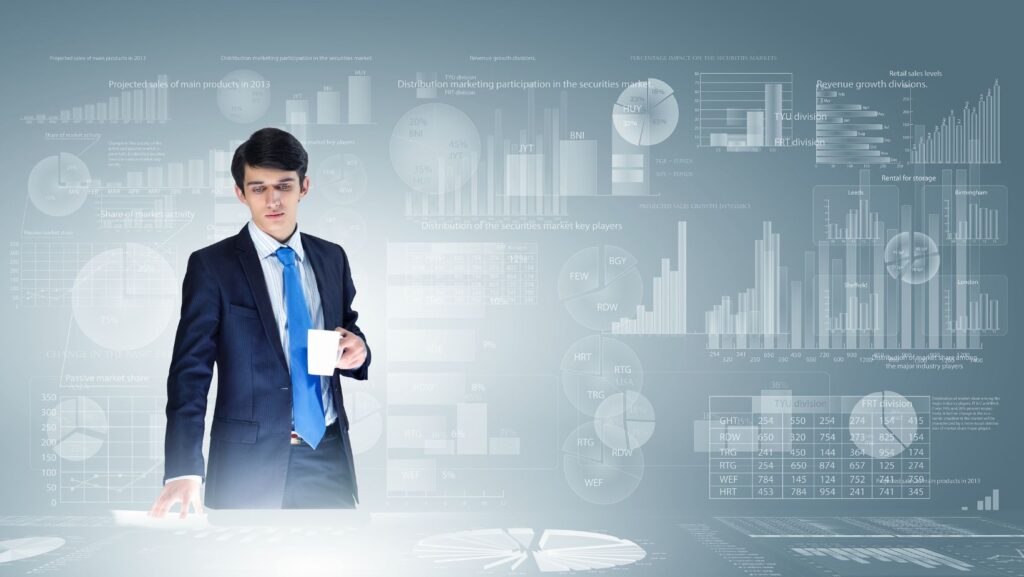
Various tools and platforms
Google Analytics: A comprehensive and widely used tool that provides insights into user behavior, conversion rates, and more.
Adobe Analytics: A powerful solution that offers advanced segmentation, real-time data, and predictive analytics capabilities.
Shopify Analytics: A built-in analytics feature for Shopify users that tracks sales, customer data, and marketing performance.
Other specialized e-commerce analytics tools: Platforms like BigCommerce and WooCommerce offer their own sets of analytics tools tailored to the e-commerce space.
Kissmetrics: A customer engagement automation platform offering behavior tracking, funnel analysis, and cohort analysis.
Mixpanel: A product analytics platform focusing on user behavior, funnel optimization, and retention analysis.
Heap: An analytics platform providing insights on user behavior, conversion optimization, and customer journey mapping.
Advanced Analytical Techniques
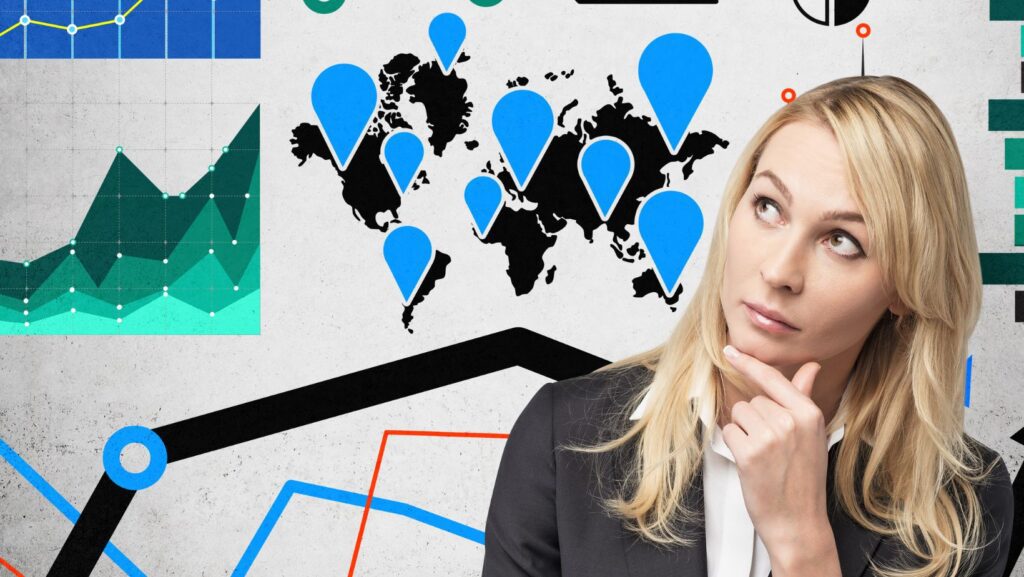
Incorporating advanced analytical techniques can provide e-commerce businesses with deeper insights and unlock new opportunities for growth and optimization.
Machine learning and predictive analytics are two such techniques that can help businesses stay ahead of the competition.
Machine Learning
Machine learning (ML) is a subset of artificial intelligence that uses algorithms to learn from data and make predictions.
By incorporating ML into e-commerce analytics, businesses can uncover patterns, trends, and insights that may be difficult to identify using traditional analytics methods. Some applications of ML in e-commerce include:
- Personalization and Recommendation Engines: ML algorithms analyze customer behavior, preferences, and past purchases to provide personalized product recommendations, creating a more engaging shopping experience that can lead to increased sales.
- Fraud Detection: ML can identify unusual patterns in customer data, transactions, or behavior, helping businesses detect and prevent fraudulent activities.
- Inventory Forecasting: ML models can analyze historical sales data, seasonality, and other factors to predict future demand for products, allowing businesses to optimize inventory levels and reduce stockouts or overstocking.
Example: Amazon uses ML-based recommendation engines to show customers related products, items “frequently bought together,” and personalized suggestions based on browsing and purchase history.
Example: E-commerce businesses can use ML algorithms to detect sudden spikes in chargebacks, unusual purchasing patterns, or multiple transactions from the same IP address, all of which may indicate fraudulent activity.
Example: E-commerce businesses can leverage ML models to forecast sales for specific products, categories, or geographic regions, enabling more efficient inventory management and reducing holding costs.
Predictive Analytics
To predict future results, predictive analytics employs historical data, statistical algorithms, and machine learning approaches. Predictive analytics can be used by e-commerce companies to plan ahead for customer behavior, improve marketing initiatives, and expedite processes.
- Customer Churn Prediction: By analyzing historical customer data and identifying patterns associated with churn, predictive analytics can help businesses identify customers at risk of attrition and proactively take steps to retain them.
- Sales Forecasting: Predictive analytics can help e-commerce businesses estimate future sales based on historical data, allowing them to make informed decisions about inventory, staffing, and marketing budgets.
- Market Trend Analysis: By analyzing trends in customer preferences, search behavior, and product performance, predictive analytics can help e-commerce businesses identify emerging opportunities and potential threats.
Example: An e-commerce business might analyze factors such as time since last purchase, frequency of support requests, and engagement with marketing emails to predict which customers are likely to churn and then target them with personalized retention campaigns.
Example: An e-commerce business could use predictive models to estimate sales for the upcoming holiday season, enabling them to adjust inventory levels, plan promotions, and allocate marketing resources effectively.
Example: An e-commerce business could use predictive analytics to identify trending products or categories, enabling them to adjust their product assortment and marketing strategies accordingly.
Integrating Analytics with Other Business Processes
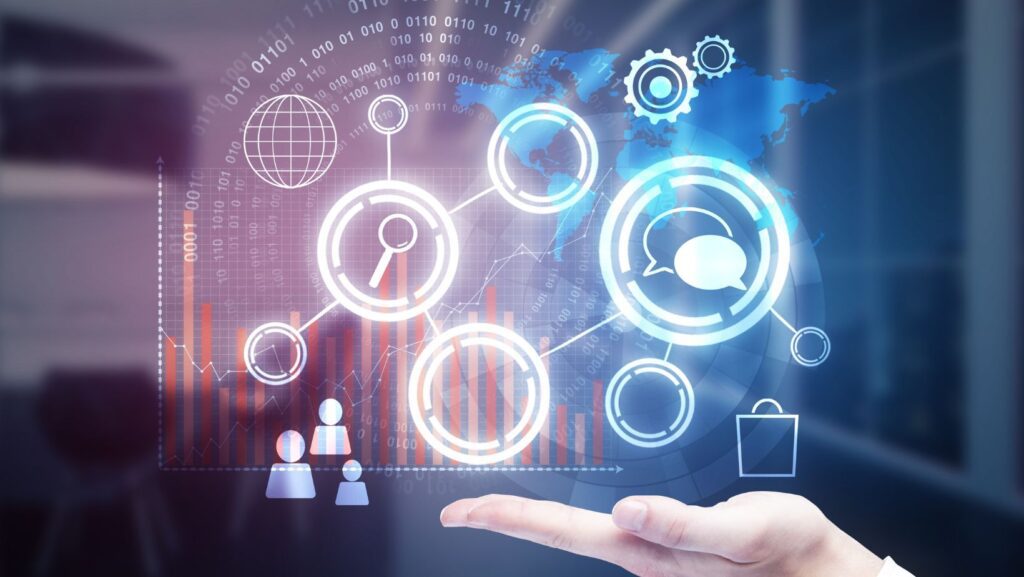
Integrating analytics insights with other business processes can provide e-commerce businesses with a more holistic understanding of their operations and drive strategic decision-making across the organization.
Supply Chain Management
- Demand Forecasting: By integrating sales analytics with supply chain management, businesses can better predict product demand, enabling them to optimize inventory levels, minimize stockouts, and reduce carrying costs.
- Inventory Optimization: Analytics can help businesses identify slow-moving or obsolete inventory, allowing them to take corrective actions, such as discounting, bundling, or discontinuing products.
Example: An e-commerce business could use sales analytics data to forecast demand for a specific product, informing decisions about reorder quantities, lead times, and supplier selection.
Example: By analyzing sales data and inventory levels, an e-commerce business can identify underperforming products and adjust their pricing or promotional strategies to improve inventory turnover.
Customer Relationship Management (CRM)
- Customer Segmentation: Integrating analytics with CRM systems allows businesses to segment their customer base based on behavior, preferences, and purchase history, enabling targeted marketing and personalized customer experiences.
- Customer Lifetime Value (CLV) Analysis: By combining purchase data with customer engagement metrics, businesses can calculate the lifetime value of their customers, informing decisions about customer acquisition and retention strategies.
Example: An e-commerce business might use analytics to identify high-value customers who frequently purchase premium products and target them with exclusive offers, loyalty programs, or personalized content.
Example: E-commerce businesses can use CLV analysis to identify the most profitable customer segments and allocate marketing resources, accordingly, focusing on acquiring and retaining high-value customers.
Product Development
- Product Performance Analysis: Integrating analytics with product development processes allows businesses to monitor product performance, identifying successful products or features and informing decisions about product improvements or new product offerings.
- Market Gap Analysis: By analyzing market trends, customer preferences, and competitor offerings, businesses can identify market gaps and develop products to address unmet customer needs or differentiate themselves from the competition.
Example: An e-commerce business could analyze sales data, customer reviews, and product return rates to determine the most popular features of a product and incorporate them into future product designs or updates.
Example: E-commerce businesses can use analytics to identify trending product categories or customer preferences and develop new products or product lines that cater to these emerging trends, providing a competitive advantage in the market.
E-commerce companies can make more data-driven decisions throughout their whole company by integrating analytics with other business processes, which leads to more effective operations and better customer experiences, which leads to more revenue and profitability.
Leveraging Data for Decision-Making
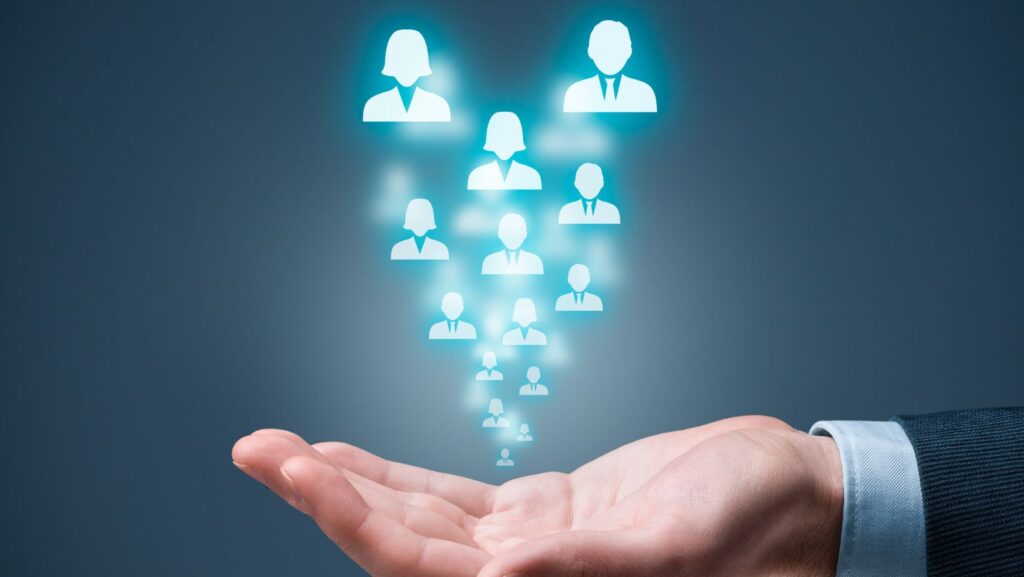
Analyzing e-commerce metrics, you can make data-driven decisions to optimize various aspects of your business.
Inventory management
Analyzing product performance: Identifying best-selling and underperforming products can help you make informed decisions about inventory levels and product assortment.
Identifying trends and seasonality: Analyzing sales data over time can reveal patterns and trends, enabling you to anticipate demand and adjust inventory accordingly.
Adjusting stock levels: Using analytics to monitor stock levels and sales velocity helps you prevent stockouts or overstocking, improving cash flow and reducing storage costs.
Marketing and advertising
Measuring campaign effectiveness by tracking key performance indicators (KPIs) such as click-through rate, conversion rate, and return on ad spend (ROAS) to evaluate the success of marketing campaigns and make necessary adjustments.
Use customer data to create targeted marketing campaigns, focusing on specific customer segments with tailored messaging, offers, and products.
Analyze the performance of different marketing channels to determine the most cost-effective methods for reaching your target audience and allocate your budget accordingly.
User experience and website optimization
Monitor user behavior metrics such as bounce rate, time on site, and conversion funnels to identify areas where users may struggle and optimize the website accordingly.
Use heatmaps, session recordings, and user feedback to pinpoint specific elements of your website that may hinder conversions or user experience.
Implement A/B testing to experiment with different design elements, calls-to-action, and content to determine which versions perform best and continuously improve your website.
Implementing a Data-Driven Culture
- To maximize the benefits of e-commerce analytics, it’s essential to cultivate a data-driven culture within your organization.
- Break down silos and encourage cross-departmental collaboration to ensure a unified approach to data-driven decision making.
- Equip your team with the necessary skills to analyze and interpret data, enabling them to make informed decisions in their respective roles.
- Set clear, measurable objectives for your business and track progress using relevant KPIs to ensure alignment and focus on achieving desired outcomes.
Example E-commerce Business
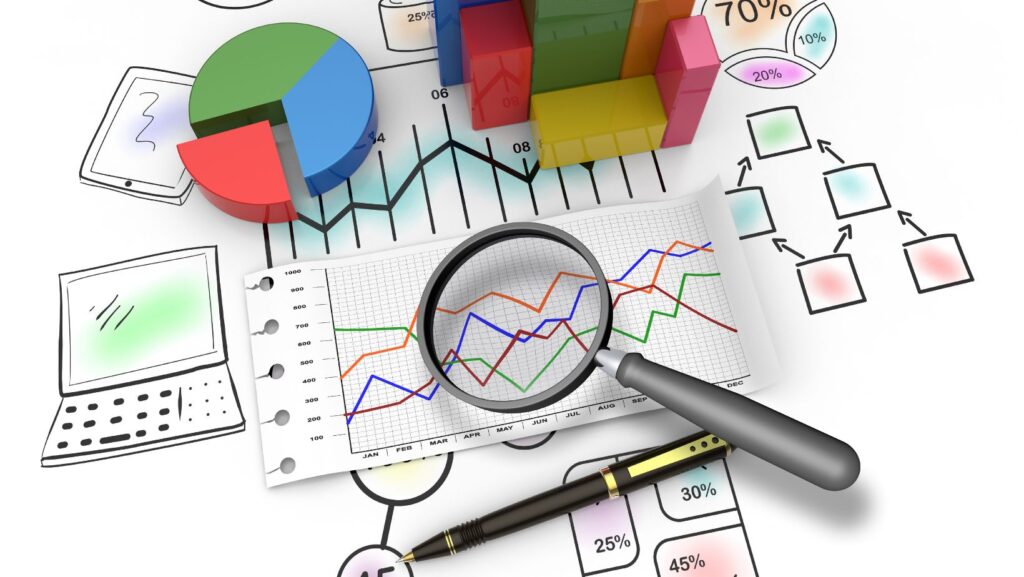
“StyleHub” is a growing online fashion retailer specializing in trendy clothing and accessories for young adults. They aim to improve their overall performance by implementing a data-driven decision-making approach using the strategies discussed in this article.
Action Plan
Analytics Tool Selection: StyleHub will start by using Google Analytics to track key metrics such as web traffic, user behavior, and conversion rates. They will also use their existing Shopify Analytics to monitor sales, customer data, and marketing performance.
Identifying Key Metrics: StyleHub’s team will identify the most relevant KPIs to track for their specific business goals, focusing on metrics such as conversion rate (industry average: 2-3%), average order value (industry average: $155), customer lifetime value, and net promoter score (industry average: 30-40).
Inventory Management
- StyleHub will analyze product performance to identify best-selling items and allocate more resources to promote and stock these products.
- They will use sales data to identify seasonal trends, adjusting inventory levels accordingly to meet demand and reduce the risk of stockouts or overstocking.
Marketing and Advertising
- StyleHub will measure the effectiveness of their marketing campaigns by monitoring KPIs such as click-through rate (industry average: 2-5%), conversion rate, and ROAS, making adjustments as needed.
- They will segment their customer base and create targeted marketing campaigns to reach specific audiences with tailored messaging and offers.
- StyleHub will allocate their marketing budget based on the performance of different marketing channels, focusing on the most cost-effective methods for reaching their target audience.
User Experience and Website Optimization
- StyleHub will analyze user behavior data to identify areas of their website that need improvement and optimize the site accordingly.
- They will use heatmaps, session recordings, and user feedback to pinpoint specific elements hindering conversions or user experience.
- StyleHub will conduct A/B testing to experiment with different design elements, calls-to-action, and content, continuously improving their website based on the results.
Implementing a Data-Driven Culture
- StyleHub will encourage collaboration between departments, fostering a unified approach to data-driven decision making.
- They will provide training and resources to team members to develop their data analysis skills.
- StyleHub will establish clear KPIs and set measurable goals, ensuring alignment and focus on achieving desired outcomes.
Key metrics available in Shopify Analytics include total sales, online store sessions, returning customer rate, average order value, and conversion rate, among others. Best practices for using Shopify Analytics include
Regularly monitoring your dashboard: Check your Shopify Analytics dashboard frequently to stay updated on your store’s performance and identify trends or potential issues.
Customizing your reports: Tailor your reports to focus on the metrics most relevant to your business objectives, making it easier to analyze and interpret the data.
Comparing performance over time: Analyze your store’s performance over different time periods to identify trends, seasonality, and growth patterns.
Integrating with other analytics tools: Connect Shopify Analytics with other analytics platforms, like Google Analytics, to get a comprehensive view of your store’s performance.
Using insights for informed decision-making: Leverage the insights from Shopify Analytics to make data-driven decisions in areas like inventory management, marketing, and user experience optimization.
Expected Outcomes
- By implementing this action plan, StyleHub aims to achieve the following outcomes
- Improved conversion rates, leading to increased sales and revenue.
- Optimized inventory management, reducing stock-related costs and enhancing product assortment.
- More effective marketing campaigns, resulting in better customer targeting and a higher return on marketing investments.
- Enhanced user experience, leading to higher customer satisfaction, increased loyalty, and more repeat purchases.
- A data-driven culture that fosters continuous improvement and empowers the team to make informed decisions.
Focusing on industry-standard benchmarks for KPIs and click-through rates, StyleHub can ensure that their performance remains competitive and that they continue to meet or exceed customer expectations.
Final Thoughts
For firms looking to expand and keep their edge in the competitive digital market, e-commerce analytics is a crucial resource.
E-commerce companies may maximize the value of their data by developing a thorough grasp of key KPIs, choosing the right technologies, and cultivating a culture of data-driven decision-making.
As a result, they can improve their tactics, streamline their business processes, and eventually achieve sustainable long-term success.
Utilizing the potential of e-commerce analytics, companies can be flexible, responsive, and adaptive while being well-positioned to take advantage of opportunities and overcome obstacles in a constantly changing environment.
Bio:
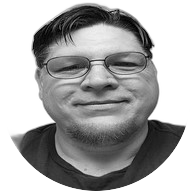
Ronnie Patterson
Ronnie Patterson, founder of MagnÜron, is a multifaceted entrepreneur with a diverse background in music, electronics engineering, and engineering management. Drawing on experience across various industries, He offers expertise in SEO, operations, and strategy to help businesses thrive. Possessing a unique perspective and unwavering commitment to collaboration, and ideal partner for growth and success.